A leader in applying advanced AI to accelerate biopharma drug discovery blending science and technology with a focus on finding solutions for complex diseases.
ABOUT US
AI-enabled drug discovery
We empower both biopharmaceutical companies and our internal scientists to harness the full potential of data and AI to accelerate the next generation of scientific advances. We have built our AI-enabled drug discovery engine to drive a revolution in drug discovery.
The Benevolent Platform™ unlocks the power of a vast biomedical data landscape to provide a multidimensional representation of human biology across all diseases.
We believe this approach will improve the probability of clinical success, and help us deliver life-changing treatments to patients – because it matters.
Progress
End-to-end drug discovery offerings
We are continuing to expand on our industry-leading collaborations further validating our drug discovery offerings and end-to-end drug discovery offerings
Multi-year collaboration with AstraZeneca continues to progress
AstraZeneca is progressing four of the most promising targets selected into the AstraZeneca portfolio in chronic kidney disease (CKD) and idiopathic pulmonary fibrosis (IPF) while good progress is being made toward further target selections in Heart Failure and SLE
Preclinical & clinical development pipeline
We are progressing five of our most advanced high-potential clinical and preclinical assets to their next inflection points. Lead asset, BEN-8744, for the treatment of ulcerative colitis is in a Phase I clinical study with topline data readout expected Q1 2024
New strategic collaboration with Merck
Multi-year collaboration to identify and develop innovative compounds, initially for three novel small molecule drug candiates in in oncology, neurology and immunology
Partner with us
We work with leading biopharma companies to discover innovative therapeutics
We partner with biopharma companies to discover novel treatments using the Benevolent Platform™. Our expertise spans across the entire drug discovery and development process and we can work on any therapeutic area or drug modality.
Latest news

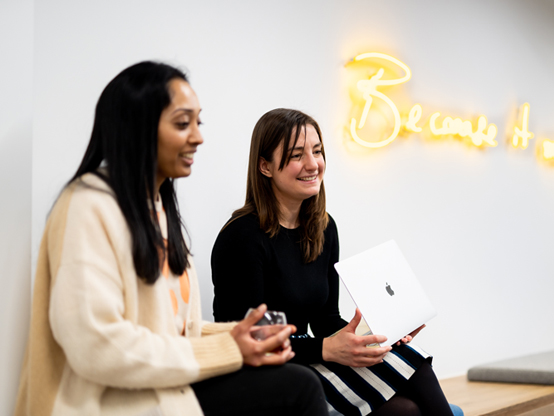
Careers
A diverse team passionate about building a healthier future
BenevolentAI embodies a new type of culture based on true collaboration between scientists and technologists who work together to redefine how drugs are discovered and developed. If you are curious and collaborative and want to help shape the future of new discoveries, we would love to hear from you.
Our offices
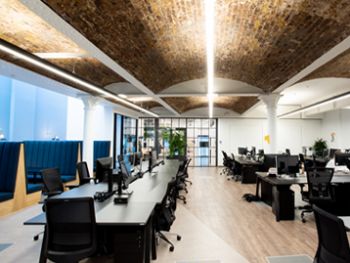
4-8 Maple Street
London W1T 5HD
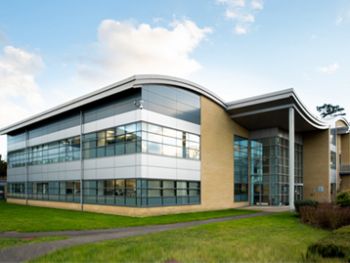
Babraham Campus
Cambridge CB22 3AT